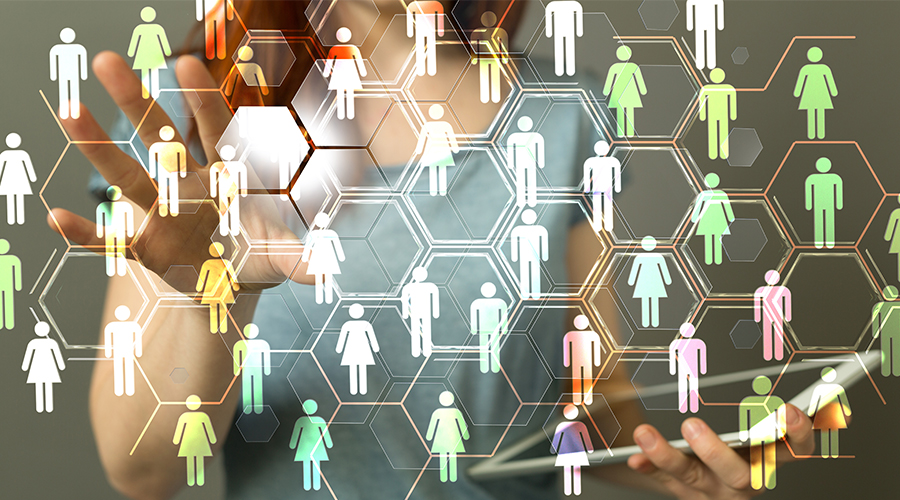
The field of computational social science (CSS) unites researchers from multiple disciplines to analyze data from social media, historical archives, and other online sources to help solve critical global issues.
Unlike the data traditionally collected by social science researchers, this digital data often contains detailed information that can provide real-time insights into human behavior and social relationships.
This comprehensive feature explains what computational social science is, provides an overview of key domains in the field, and explores four practical applications of CSS that could help improve lives worldwide.
What is computational social science?
Computational social science represents an intersection of computer science and the social sciences, including political science, economics, sociology, psychology, and anthropology.
Technological advances have resulted in a massive volume of digital data continuously generated by online apps and social media platforms. Social science researchers trained in data analysis and programming can use this information to address complex problems such as socioeconomic inequalities, political division, and crime.
The computational tools and methods used in CSS include:
- Data mining
- Data visualization
- Web scraping
- Text analysis
- Machine learning
- Natural language processing (NLP)
Domains of computational social science
Without a strong background in computer science, it can be difficult to navigate the different facets and themes in the field of computational social science. Below are summaries of some of the key spheres of CSS.
Computational economics
In computational economics, computer scientists and economists use software and other computational tools to solve analytical problems and make more accurate predictions about the economy and financial markets.
Examples of the data analysis methods employed in computational economics include agent-based computational modeling and computational modeling of dynamic macroeconomic systems.
Social network analysis
Social network analysis is a theoretical approach and set of methods investigating complex social systems based on relationships, structures, location, roles, connectivity, matrix representations, and other network properties.
Key concepts in social network analysis include:
- Homophily: The tendency for people to seek out those similar to themselves
- Social contagion: The rapid spread of attitudes, emotions, and behaviors throughout groups without reason
- Social capital: The theory that interpersonal relationships can create value
Cliodynamics
Cliodynamics is an interdisciplinary research area that integrates historical macro sociology, economic history, cultural and social evolution, long-term mathematical modeling, and the construction and analysis of historical databases to make predictions regarding issues such as social and political unrest, war, and violence.
The goal is to apply analytical, predictive scientific methods to social sciences (especially history) to identify patterns in social trends and forces that have continuously shaped society. However, the field is viewed with skepticism by many academic historians.
Culturomics
In the sub-field of culturomics, computational social science researchers study human behaviors, linguistic phenomena, and cultural trends by analyzing vast amounts of textual data from Google Books or other digital sources.
For instance, research that analyzed unique common words in the English lexicon in 1900, 1950, and 2000 found that in the latter year, more unique words were used in text than ever before. While studying the nature of celebrity over time, researchers found that people become more famous at an earlier age but are also forgotten at a faster rate.
CSS analytics: the role of X and digital media
Whenever users visit social media platforms such as X or other online media, they leave a digital footprint, including text, images, interaction metrics, and metadata that can be analyzed to make predictions about human social behavior. Researchers can combine these massive data sets with randomized survey responses that help them better understand human culture, including topics like how information spreads across networks.
For example, research on the viral spread of social media messages about autism spectrum disorders found that exposure to emotional language makes users more likely to become emotional themselves and identify with emotional content. Additionally, the use of angry language can contribute to the viral dissemination of misinformation.
Applying CSS and data analysis to 4 real-world problems
As mentioned above, melding data science techniques with social science research can lead to a much greater understanding of human social phenomena to help address real-world issues. Below are four such ways that computational social science tools are being used.
1. Supporting public health research
The goal of the field of public health is to improve the health of individuals and their communities. Public health professionals educate people on how to adopt healthy lifestyles, administer services, conduct disease research, and recommend policies to governments.
Computational social science research has many exciting applications to help bolster public health efforts. For example, members of the CSS Lab at the University of Pennsylvania used mobility data related to COVID-19 to conduct research and train epidemiological models to predict the impact of policies related to vaccination and reopening efforts during the pandemic.
More broadly, the Annual Sociology Review cites research by demographers who used digital data from Google Search and Facebook to analyze public health issues, including the prevalence of selective abortion in India, rates of binge drinking at US colleges, lifestyle disease in 47 countries, and patterns in fertility, mortality, and migration.
Computational health science is an emerging sub-field of CSS that harnesses advanced machine learning and graphical network-based analytics to provide insights into biological processes, clinical decision-making support, and the discovery of novel drugs and treatments. These efforts could have a significant impact on future public health outcomes.
2. Reducing political polarization and radicalization
There’s no question that political unrest and division is a major problem in the United States and abroad. According to an article in Greater Good Magazine, the many adverse effects of political polarization include:
- Increased community segregation by political party
- A more antagonistic political culture
- A loss of trust in key institutions
- An inability to come to a consensus on critical issues such as gun control
- A rise in hate crimes and intergroup violence
In recent years, numerous scientific studies have employed computer science methods to analyze large data sets related to political science. Examples detailed in the Annual Sociology Review include:
- Using Google Search data to study the demographic factors that can increase susceptibility to violent radicalization
- Examining how elites in journalism, politics, and entertainment help shape global political discourse and drive the polarization of views
- Analyzing data from social media and other platforms to better understand how digital tools have played a role in protests and other collective political action
- Using online data, agent-based modeling, automated text analysis, and network-based research to study political tribalism and extremism, homophily, and the formation of “echo chambers” that limit exposure to opposing ideas
- Performing experiments to learn how partisans can change their beliefs. Some findings include that exposure to opposing political views can increase partisanship while using specific moral language and matching linguistic styles may help reduce it.
3. Understanding perceptions around climate change
A 2023 report from UN Climate Change found that intergovernmental climate action plans are not effective enough to limit the rise of global temperatures to 1.5 degrees Celsius or meet the goals of the Paris Agreement. Greenhouse gas emissions need to be cut by 43 percent by 2030 to prevent dire impacts such as more frequent and harsh heatwaves, droughts, and rainfall.
According to Pew Research, perceptions in the US are polarized between the two major political parties, with nearly 78 percent of Democrats viewing climate change as a major threat compared to just 23 percent of Republicans. Climate change also falls in the top half of priority issues for Democrats and ranks second to last for Republicans.
A study using large-scale computational data and methods explored how the polarization of climate change politics in the US is influenced by a network of political and financial groups that actively work against climate change initiatives. The research found organizations with corporate funding were more likely to have created and amplified texts designed to polarize climate change issues, ultimately influencing public discourse.
In 2019 and 2020, Australian bushfires caused 33 human deaths, the displacement or death of close to 3 billion animals, and a devastating loss of habitat. This destruction attracted global public attention, and computational social science researchers sought to better understand the connection between the bushfires and public perceptions surrounding climate change.
Researchers used a data set of 9,000 tweets to identify and track keywords and hashtags to analyze perceptions of causality, blame, urgency, and prevention tactics related to the bushfires. Their results provided valuable insights; despite the spread of some forms of disinformation, X activity seemed to strengthen support for climate change action.
4. Improving insights into global poverty
According to the United Nations, the global poverty rate is expected to reach 7 percent by 2030, and many of the world’s vulnerable populations in low-income countries are not covered by social protections. Additionally, violent conflicts and the increasing impacts of climate change make it more difficult to combat poverty around the world.
Although industrialized nations have a plethora of demographic big data, one of the critical challenges to fighting poverty in the developing world is the lack of national statistical systems to identify location and socioeconomic status.
To help address this lack of data, CSS methods have been used in the following ways:
- Scientists studied metadata from call logs of residential mobile phone users in Rwanda, analyzed their locations, and implemented a randomized survey to create a model to estimate poverty levels of households, regions, or entire nations
- Researchers analyzed spatial data from Open Street Maps and the European Space Agency’s land use cover with five machine learning algorithms to predict poverty levels in Medellín, Colombia, which provides policymakers with a tool to make poverty and social interventions
- Scholars confirmed that wealth and poverty estimates derived from three machine learning techniques outperformed those using the traditional linear mixed model
Models compiled by computational tools can be used by legislators and nonprofit organizations to better target humanitarian aid efforts in areas experiencing higher levels of poverty and use them to help assess other social inequalities in gender, race, ethnicity, health, and education.
Ready to enhance your digital literacy?
The Data Analytics and Psychological Sciences concentration for the Bachelor of Applied Arts and Sciences (BAAS) at Penn LPS Online explores the intersection of data analysis and the science of well-being.
In this liberal arts program, you’ll learn how well-being is measured, what contributes to human flourishing, and how to apply analytical and statistical methods to interpret and communicate data.
This Ivy League program prepares you to:
- Understand advanced predictive modeling and machine learning
- Implement and analyze basic regression models
- Design surveys and experiments, including A/B tests
- Develop data analysis in R and statistical programming skills
- Explore key research themes in positive psychology and their relevance
- Investigate applications of positive psychology in business, healthcare, education, and the nonprofit sector
If you haven’t already, apply to Penn LPS Online today and enroll in the Data Analytics and Psychological Sciences concentration for the BAAS degree. You can also view our course guide to learn more about what’s available in any upcoming term.
Additional sources